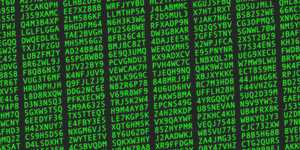
GUID and UUID in SAP HANA
26. Januar 2022
You will often encounter GUID and UUID in SAP HANA and in S/4HANA as key fields. As long as these do not have to be interpreted or…
26. Januar 2022
You will often encounter GUID and UUID in SAP HANA and in S/4HANA as key fields. As long as these do not have to be interpreted or…
25. Januar 2022
In this blog post, I would like to share a small example of an AMDP aka. HANA Script or AMDP Script ((Actually there is no HANA Script or…
14. September 2021
The HANA Web-Console (HWC) from Brandeis Consulting is the quick entry into SQLScript programming on the SAP HANA database . For trainings…
19. Juli 2021
The 2nd edition of the English edition of SQLScript for SAP HANA is scheduled for release on August 26, 2021. The issue is updated and…
16. Januar 2021
SAP has unfortunately decided to stop making its VM images of the free HANA Express , which were previously available in the cloud from…
29. Dezember 2020
Cheat Sheet - The SAP HANA SQLScript Overview This SQLScript Overview is the perfect complement to the training of Brandeis Consulting…
29. Oktober 2020
When loading data from source systems, there are requirements in SAP BW that are often repeated at the field level. This includes, above all…
16. August 2020
The Neo environment of the SAP Cloud Platform will be switched off on November 14th! This also means that a popular opportunity to create a…
Informationen zur SAP HANA Datenbank.
Copyright © 2024 Brandeis Consulting